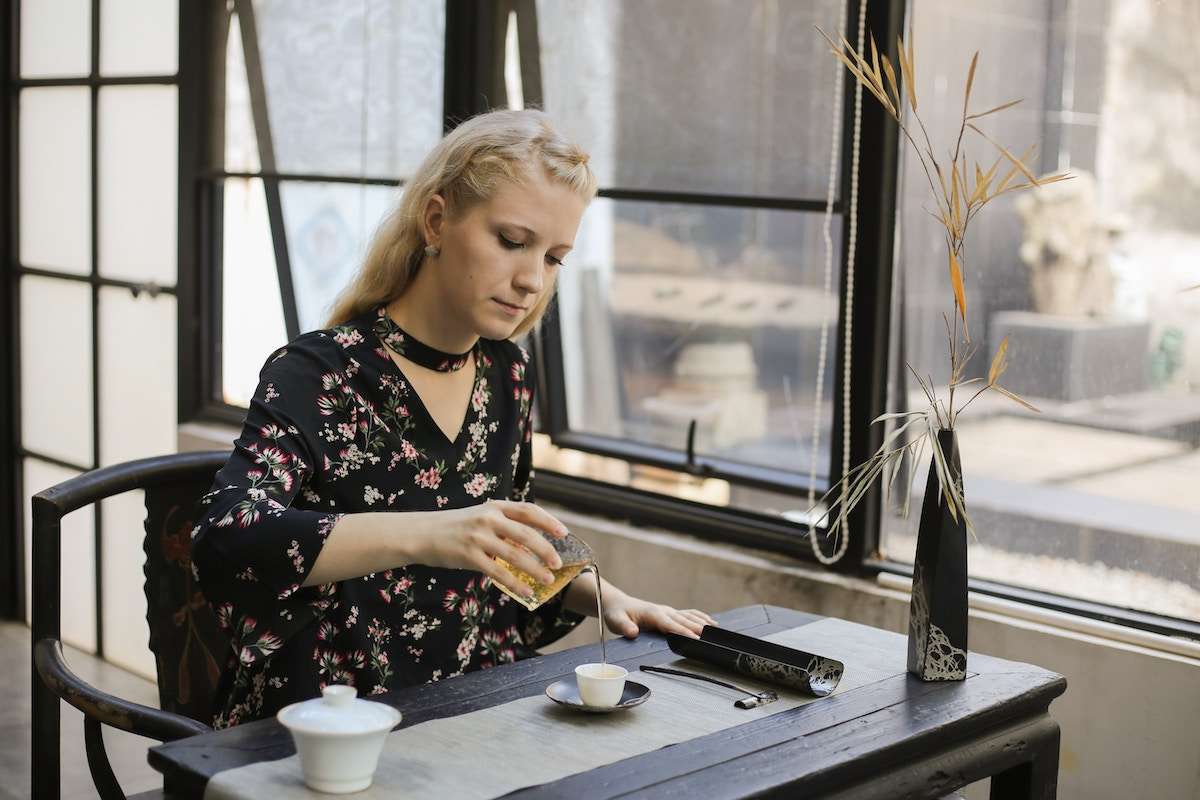
The Design of Experiments - Using Statistics and Probability
This article is my understanding of guidelines for designing experiments using statistics and probability as a way of judging results which were laid out by Sir Ronald Aylmer Fisher, a British statistician and geneticist. He has been described as "a genius who almost single-handedly created the foundations for modern statistical science and single most important figure in 20th century statistics."
As an example, he told the story of a lady who claimed to taste the difference between milk poured into her tea and tea poured into her milk. Fisher considered ways to test that. What if he presented with just one cup to identify?
If she got it right one time, you'd probably, well yeah, but she had 50-50 chance just by guessing, of getting it right. So you'd be pretty unconvinced that she has the skill. Fisher proposed that a reasonable test of her ability would be eight cups.
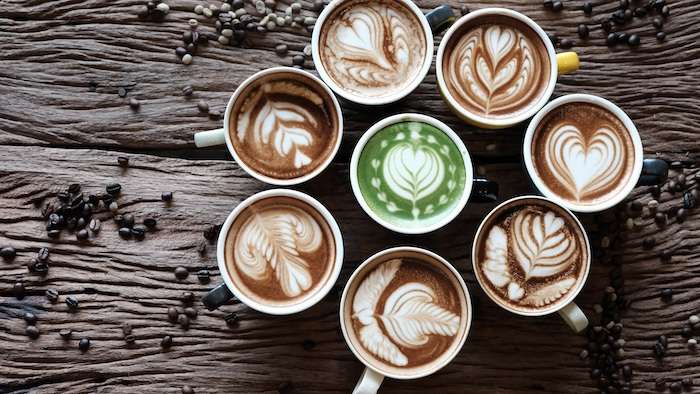
Why eight? Because that produced 70 possible combination of the cups, but only one with them seperated correctly. If she got it right, that wouldn't prove she had a special ability but Fisher could conclude, if she was just guessing, it was an extremely unlikely result, a probability of just 1.4%.
Thanks mainly to Fisher, that idea became enshrined in experimental science as the
p-value { p for probability }
If you assume your results were just due to chance, that what you were testing had no effect, what's the probability you would see those results or something even more rare?
If you assume that there's a process that is completely random, and you find that it's pretty unlikely to get your data, then you might be suspicious that something is happening. You might conclude in fact that it's not a random process. That it's interesting to look at what else might be going on, and it passes some kind of sniff test.
Fisher also suggested a benchmark.
Only experimental results where the p-value was under 0.05, a probability of less that 5%, were worth a second look.
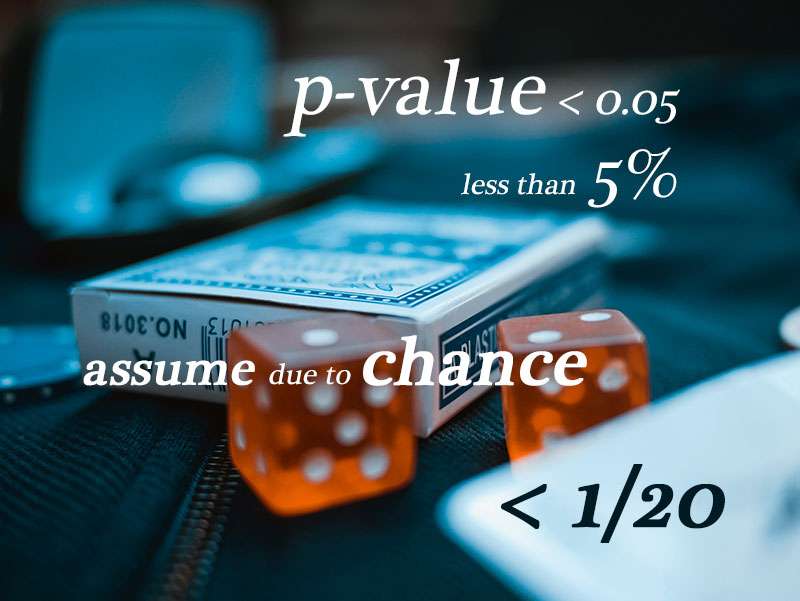
Not very likely.
He called those results "statistically significant".
In statistical hypothesis testing, a result has statistical significance when it is very unlikely to have occurred given the null hypothesis. Ronald Fisher advanced the idea of statistical hypothesis testing, which he called "tests of significance", in his publication Statistical Methods for Research Workers.
Since then, p-values have been used a convenient yardstick for success by many, including most scientific journals. Since they prefer to publish successes, and getting published is critical to career advancement, the temptation to massage and manipulate experimental data into a good p-value is enormous. And it has a name too. P-hacking.
Comments